Much ado about the demise of nudging
- Elina Halonen
- Nov 11, 2022
- 15 min read
Updated: Sep 24, 2024
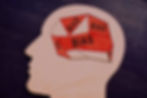
I have been meaning to write about the nudge meta-analysis for several months, but since I had quite a few things to say, I kept putting it off. A subtle nudge from someone on LinkedIn finally prompted me to arrange my thoughts.
There have been many commentaries on the paper already that are worth reading:
Making Sense of the “Do Nudges Work?” Debate (Michael Hallsworth)
Moving behavioral science beyond the nudge and into the private sector (Scott Young)
Realistic Reasons to be Bullish on Nudging (Ed Bradon)
Evidence for behavioural interventions looks increasingly shaky (The Economist)
No evidence for nudging after adjusting for publication bias (PNAS)
In a nutshell, I agree with Michael Hallsworth's conclusions that:
The real situation is more complicated, interesting, and hopeful than this wild oscillation between “big effects” and “no effects” suggests. -- We have a simplistic and binary “works” versus “does not work” debate. But this is based on lumping together a massive range of different things under the “nudge” label, and then attaching a single effect size to that label.
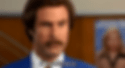
And I fully agree with Scott Young that "using nudging as an entry point has been somewhat of a double-edged sword" because for 10 years it has led many to view behavioral science as either gimmicky party tricks or something that anyone can quickly learn and apply themselves. As Scott points out, the enthusiastic but haphazard application of BeSci concepts based on pop science books often leads to uneven or unmeasurable results, and eventually to organizations becoming sceptical or abandoning efforts when they are unable to see a clear outcome or ROI. In these situations, it's nearly impossible to convince someone that there is actual rigorous science behind BeSci - and it's often especially difficult to convince them to use "BeSci as a lens", as Scott suggests.
For me, BeSci is both a lens and a tool:
a lens to quickly, effectively and objectively identify patterns for problems that can be understood better with BeSci knowledge AND to diagnose the challenge in behavioural terms
a tool to either directly design psychological solutions OR optimise other kinds of solutions (e.g. changing the environment) with the help of psychological knowledge
Part of the problem in this nudge debate is that for almost 10 years we have sold BeSci as a tool in a way that is like conceptual hyperbolic discounting by promising quick, painless benefits now. Changing the discussion sometimes feels like trying to turn around the Titanic that is well on its way towards the iceberg of oblivion and irrelevance. For example, I recently analysed keywords that drive organic traffic to the websites of a dozen BeSci consultancies and the top ones were a laundry list of heuristics and biases.
It is also often difficult to manage clients' or stakeholders' expectations of the quick, easy tweaks with disproportionate returns (the "BeSci Magic" they've read about) when you know that talking about rigorous science will instantly dampen their enthusiasm... and encourage them to find another consultant who is more fun.


Going back to the discussion around the demise of the nudge, I think there are several things we need to think about before drawing any conclusions about the PNAS paper - all of which I will address below in detail:
Unclear definition of what nudging is
Lack of unified theory
The importance of context and subroups
Methodological and statistical questions around the meta-analysis
I make no promises to be entertaining, but I have tried to lighten up the mood when you get to the extra-sciencey part with statistics and methodological concerns. For the rest, a strong coffee may be helpful!
What is nudging anyway?
Definitions matter - if you don't really understand the concept of nudge, you might try to apply it in the wrong place and then be disappointed when it doesn't work. Yet we're not surprised using a hammer doesn't help us fix a screw!
The original definition of a nudge by Thaler and Sunstein (2008) goes as follows:
A nudge... is any aspect of the choice architecture that alters people’s behavior in a predictable way without... significantly changing their economic incentives.
On the surface it seems straightforward - the latter part of the definition (without economic incentives) has been considered crucial for defining what constitutes a nudge. In contrast, you could also classify interventions as nudges or not based on the decision-making process, rather than on the motive behind the intervention like Thaler and Sunstein do. For example, we could use this definition by Löfgren & Nordblom (2020):
“a nudge is an alteration of the inattentive choice situation, which would not affect the attentive choice.”
This definition of a nudge is some change in the situation where a choice is made inattentively – but not if the choice was made attentively. Therefore, for a behaviour to be susceptible to nudging, the choice has to be made inattentively.
A nudge is more likely to be effective if the choice is not very important to the person – in other words, the consequences of getting the choice wrong are not meaningful to them in some way. Nudges are less likely to have any effect at all for choices that someone considers to be important, and other kinds of intervention should be considered (e.g. information, regulations or price).
This is just one conceptualisation of a nudge, but I'm sharing it as an illustration of how in-built assumptions can influence what kinds of nudges people will attempt to create and test. As a consequence, the application situations will also vary wildly and ultimately that will influence the potential success of an intervention. Therefore, we should not be surprised that there's a lot of variation in the effects of nudge interventions - instead, we should expect it because when it comes to behavioural interventions, the devil is in the details.
To be honest, I often find the discussion about nudges frustrating because it's a very limited perspective into human decision-making.
The basic premise of the nudge approach is the concept of bounded rationality - that human beings are fallible, poorly informed as well as suffering from myopia, inertia and self-control issues. In other words, human decision making is full of somewhat predictable flaws and consequently the scientific field should focus on studying them and the applied world on fixing them.
Over the past 40 years, the heuristics and biases program (H&B) has uncovered a lot of systematic violations of reasoning and decision making which have led to a loose consensus that humans have innate cognitive limitations which lead us to instinctively rely on heuristics. This insight was originally the core idea of nudging: that the policy makers could leverage people's cognitive and motivational inadequacies as a way to steering them to better decisions for their own long-term welfare - ones that are aligned with their ultimate goals.
However, it's not the only view on how humans behave and decide - it has received plenty of criticism like everything in academia - something that is often missed in the conversations on the applied side of practitioners. In fact, the research on heuristics and biases was criticized early on in the 80s as the "psychology of first impressions" and that there is more to human decision making and problem solving than their first response in a given situation.
Read more:
Lack of attention on theory, context and subgroups/individual differences
Despite what many people think, "nudge" (or nudging) is not a specific framework or even one cohesive theory.
Instead, it's better seen as a collection of techniques and approaches with shared characteristics for designing choice environments in a way that changes behaviour. Think of a power tool with different bits for different jobs! As such, it doesn't help us understand more detailed dynamics of nudges - for that, we need something more structured. With a causal explanatory approach we could construct scenarios of potential outcomes and determine what features of an intervention could influence them.
As a great paper on how behavioural interventions can fail suggests, these questions are helpful to ask when planning an intervention:
What factors could be causally relevant to the success of the intervention?
How could the intervention influence these factors?
What precautionary measures should be taken to avoid failure?

One key problem is that a lot of the "nudging literature" ignores context: every behavioural challenge is a causal structure extending way beyond the immediate choice architecture - not analysing problem in its environment limits the impact of an intervention. Thinking about what factors in the wider environment are contributing to a behaviour is a crucial starting point for identifying interventions and tailoring them to the problem.
Unfortunately, behavioural economics research has been focused on the particular set of options that an individual decision maker faces without acknowledging or explicitly specifying the environment in which the choice architecture is embedded. To me, it should be obvious that the success of a behavioural intervention can critically depend on societal and cultural factors as well as more tangible environmental factors that constrain the consequences of a decision-maker's choices so we should aim to determine what kind of environment the behavioural challenge is embedded in, and whether nudges are likely to be effective. We can follow a process of asking specific questions to identify environmental factors that are critical to the success of intervention
One important point is that subgroups matter: depending on the situation they can result in the intervention not working at all, backfiring or resulting in unexpected side effects. As obvious as this might seem, it's not been a big part of the discourse in behaviour change or behavioural insights so far, and in some cases this issue has even been somewhat brushed under the carpet because it doesn't quite fit the initial promise of small changes resulting in big effects that nudging was saddled with.
Many behavioural interventions are designed to target the largest number of people in one go as possible - after all, the promise of behavioural economics and nudging is that small changes can have big effects. This implicit universalist premise has long been "supported" by the lack of conclusive research evidence on individual differences in decision-making and as a consequence the question of whether subgroups might need to be considered has largely been ignored.
This assumption of sufficient homogeneity of behaviour and especially its underlying mechanisms has come at the expense of being sensitive to varying backgrounds, values and preferences of a particular population. In reality, effective behaviour change needs different interventions or combinations of them to deal with the same issue in different subgroups of the population.
One reason for this lies in the incentives built into the system that produces the academic literature. A lot of foundational research has been focused more on discovering so-called main effects because:
They're easier to publish in journals because they tell a simpler story
Novel contribution to the literature is also easier to demonstrate to journal editors
Novel contributions are also boost people's careers more also leads to researchers chasing new phenomena to coin (think "fresh start effect") instead of exploring the nuance of things like different contexts or subgroups (i.e. interaction effects, hidden confounds and individual differences)
To leverage the authority bias, here's Dan Ariely:
The biggest challenge for our field in the next 10 year would be to understand the generality of the findings we have. We have lots of findings and different aspects and we have assumed for a long time that they would just carry over in different contexts and different occasions. Of course, when we talk about the theory of mind or psychology, the context doesn’t have to be part of the theory, but as we get access to more people and more cultures, I think we’ll have to have a more nuanced understanding of our theories and we will have to learn to adjust them based on other intervening factors that might come from culture for example. We have been ignoring culture too much. It has less to do with the theory and more to do with application: as we try to apply things and try to change human behavior, we will need to understand those nuances to a larger degree.
Other issues include academic siloes that result in a narrow theory-base, the messy and complex nature of reality and the lack of a cumulative theoretical frameworks - as Muthukrishna and Henrich (2019) note in their Nature article "A Problem In Theory":
Without an overarching theoretical framework that generates hypotheses across diverse domains, empirical programs spawn and grow from personal intuitions and culturally biased folk theories. By providing ways to develop clear predictions, including through the use of formal modelling, theoretical frameworks set expectations that determine whether a new finding is confirmatory, nicely integrating with existing lines of research, or surprising, and therefore requiring further replication and scrutiny. Such frameworks also prioritize certain research foci, motivate the use diverse empirical approaches and, often, provide a natural means to integrate across the sciences.
The behavioural science industry has traditionally oriented itself (albeit loosely) around the area of behavioural economics, relying on the work of cognitive psychology to identify ‘biases’ in human behaviour and seek to use nudges as solutions to help deliver positive outcomes. Collins suggests that the underlying theoretical basis for behavioural scientists is the rational-actor model of economics in which people make decisions based on their preferences and the constraints that they face. Behavioural economics offers the many deviations from this rational-actor model but in doing so, de-facto retains it at the heart of its discipline. In this way, biases are the equivalent of the deviations that astronomers used to protect what was increasingly apparent, that the underlying model was simply wrong.
Then there is a more fundamental question about the limitations experimental methods used in the theories that nudges are based on: not everything that can be measured matters, nor can everything that matters be (easily) measured.
Read more
All that glitters is not gold - 8 ways behaviour change can fail
Please, Not Another Bias! The Problem with Behavioral Economics (Jason Collins)
Please not another bias: correcting the record (Jason Collins)
Please not another bias: Take two (Jason Collins)
How big is the effect of a nudge? (Jason Collins)
Going beyond the obvious (Colin Strong)
Is behavioural science using the wrong model? (Colin Strong)
Replication Schmeplication? The State of Behavioral "Science" (Michael Inzlicht on "It's all a bunch of BS" podcast)
Reckoning with the past, Too Soon and Updating beliefs (Michael Inzlicht)
Is There a Generalizability Crisis? (Two Psychologists, Four Beers podcast)
Let's not forget the methodological angle!

Some of the details of the statistical critiques are beyond my skillset but from reading the various analyses of the paper I've concluded a few things.
(I also thought the stats section needed gifs to motivate people to keep reading, even if using them outs me as a millennial.)
Data Colada notes two findamental problems with meta-analytic averages that should be enough to concern anyone even if you're not a stats whiz.
1. Some Studies Are More Valid Than Others
Meta-analysis has many problems. For example, meta-analyses can exacerbate the consequences of p-hacking and publication bias and common methods of correcting for those biases work only in theory, not in practice. (Data Colada)
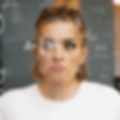
Some studies in the scientific literature have clean designs and provide valid tests of the meta-analytic hypothesis. Many studies, however, do not, as they suffer from confounds, demand effects, invalid statistical analyses, reporting errors, data fraud, etc. (see, e.g., many papers that you have reviewed). In addition, some studies provide valid tests of a specific hypothesis, but not a valid test of the hypothesis being investigated in the meta-analysis. When we average valid with invalid studies, the resulting average is invalid. (Data Colada)
More fundamentally, there are also the issues of publication bias and selection bias which inevitably also impact a meta-analysis like this:
I’m concerned about selection bias within each of the other 200 or so papers cited in that meta-analysis. This is a literature with selection bias to publish “statistically significant” results, and it’s a literature full of noisy studies. If you have a big standard error and you’re publishing comparisons you find that are statistically significant, then by necessity you’ll be estimating large effects. This point is well known in the science reform literature. Do a meta-analysis of 200 studies, many of which are subject to this sort of selection bias, and you’ll end up with a wildly biased and overconfident effect size estimate. It’s just what happens! Garbage in, garbage out. (Gelman)
I'm also concerned that the analysis includes 11 papers by Brian Wansink, whose integrity as a researcher has been called into question and who has had numerous papers retracted. It's especially concerning as the authors note in the paper that"food choices are particularly responsive to choice architecture interventions, with effect sizes up to 2.5 times larger than those in other behavioral domains" - perhaps influenced by the number of papers in the food choice literature from a scientist whose research has been known to have serious issues.
Large huge effect size estimates are not an indicator of huge effects; they’re an indication that the studies are too noisy to be useful. And that doesn’t even get into the possibility that the original studies are fraudulent. (Gelman)
I can't help but wonder what else has been included that isn't entirely trustworthy science especially as the paper also includes the now-infamous and retracted Shu et al (2012) paper - aka the Dan Ariely Scandal.
2. Combining Incommensurable Results
Averaging results from very similar studies – e.g., studies with identical operationalizations of the independent and dependent variables – may yield a meaningful (and more precise) estimate of the effect size of interest. But in some literatures the studies are quite different, with different manipulations, populations, dependent variables and even research questions. What is the meaning of an average effect in such cases? What is being estimated? (Data Colada)
For example, I'm not convinced it's sensible to report an aggregate effect size involving any study that sits under the banner of “choice architecture” or a “nudge” (like Jason Collins notes). For example, the cultural and legal context of some of the organ donation studies included is vastly different.
Given the credentials of the authors of Data Colada, I comfortable in trusting their assessment of the PNAS nudging meta-analysis and I'll copy some of their key points here - if it seems like a lot to read, keep in mind that the actual blog post is much longer...
Imagine someone tells you that they are planning to implement a reminder or a food-domain nudge, and then asks you to forecast how effective it would be, would you just say d = .29 or d = .65 and walk away? Of course not. What you’d do is to start asking questions. Things like, “What kind of nudge are you going to use?” and “What is the behavior you are trying to nudge?” and “What is the context?” Basically, you’d say, “Tell me exactly what you are planning to do.” And based on your knowledge of the literature, as well as logic and experience, you’d say something like, “Well, the best studies investigating the effects of text message reminders show a positive but small effect” or “Giving people a lot less to eat is likely to get them to eat a lot less, at least in the near term.” What you wouldn’t do is consult an average of a bunch of different effects involving a bunch of different manipulations and a bunch of different measures and a bunch of a different contexts. And why not? Because that average is meaningless. (link)
In the commentary claiming, in its title, that there is “no evidence” for the effectiveness for nudges, the authors do acknowledge that “some nudges might be effective”, but mostly they emphasize that after correcting for publication bias the average effect of nudging on behavior is indistinguishable from zero. Really? Surely, many of the things we call “nudges” have large effects on behavior. Giving people more to eat can exert a gigantic influence on how much they eat, just as interventions that make it easier to perform a behavior often make people much more likely to engage in that behavior. For example, people are much more likely to be organ donors when they are defaulted into becoming organ donors. For the average effect to be zero, you’d either need to dispute the reality of these effects, or you’d need to observe nudges that backfire in a way that offsets that effects. This doesn’t seem plausible to us. (link)
It is also worth thinking about publication bias in this domain. Yes, you will get traditional publication bias, where researchers fail to report analyses and studies that find no effect of (usually subtle) nudges. But you could imagine also getting an opposite form of publication bias, where researchers only study or publish nudges that have surprising effects, because the effects of large nudges are too obvious. For example, many researchers may not run studies investigating the effects of defaults on behavior, because those effects are already known. (link)
If you just skimmed all of that, here's the important part in plain English:
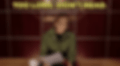
In sum, we believe that many nudges undoubtedly exert real and meaningful effects on behavior. But you won’t learn that – or which ones – by computing a bunch of averages, or adjusting those averages for publication bias. Instead, you have to read the studies and do some thinking. (link)
Read more
How big is the effect of a nudge? (Jason Collins)
Meaningless Means: Some Fundamental Problems With Meta-Analytic Averages (Data Colada)
Meaningless Means #1: The Average Effect of Nudging Is d = .43 (Data Colada)
This meta-analysis of nudge experiments is approaching the platonic ideal of junk science (Andrew Gelman)
A Credibility Crisis in Food Science (The Atlantic)
Where next for nudging?
It feels like we are on the verge of a new, more honest era for nudging and behaviour change after a decade of unbridled optimism and enthusiastic marketing.
First, we should look at the common cognitive and behavioural characteristics of failed interventions - what can we relate back to cognitive and social theories of behaviour?
Second, how can we best advance the theoretical and methodological foundations of behaviour change research?
However, as a practitioner it's beyond me to propose tangible solutions - let alone do something to solve this problem. Instead, I think we need more holistic and thorough diagnoses of behavioural challenges combined with logic models and theories of change like those used in implementation science - even if they require more effort than implementing simple nudges and are far less exciting or "pop science book friendly".
And ultimately, like Michael Hallsworth says:
A much more productive frame is to see behavioral science as a lens used for wide-ranging inquiries, rather than a specialist tool only suitable for certain jobs.
And on that note, if you want to take a look at the breadth of BeSci applications there's now a new repository of case studies, application examples and learning resources - check it out here!
